Email Spam Detection Using Machine Learning: Enhancing Business Security
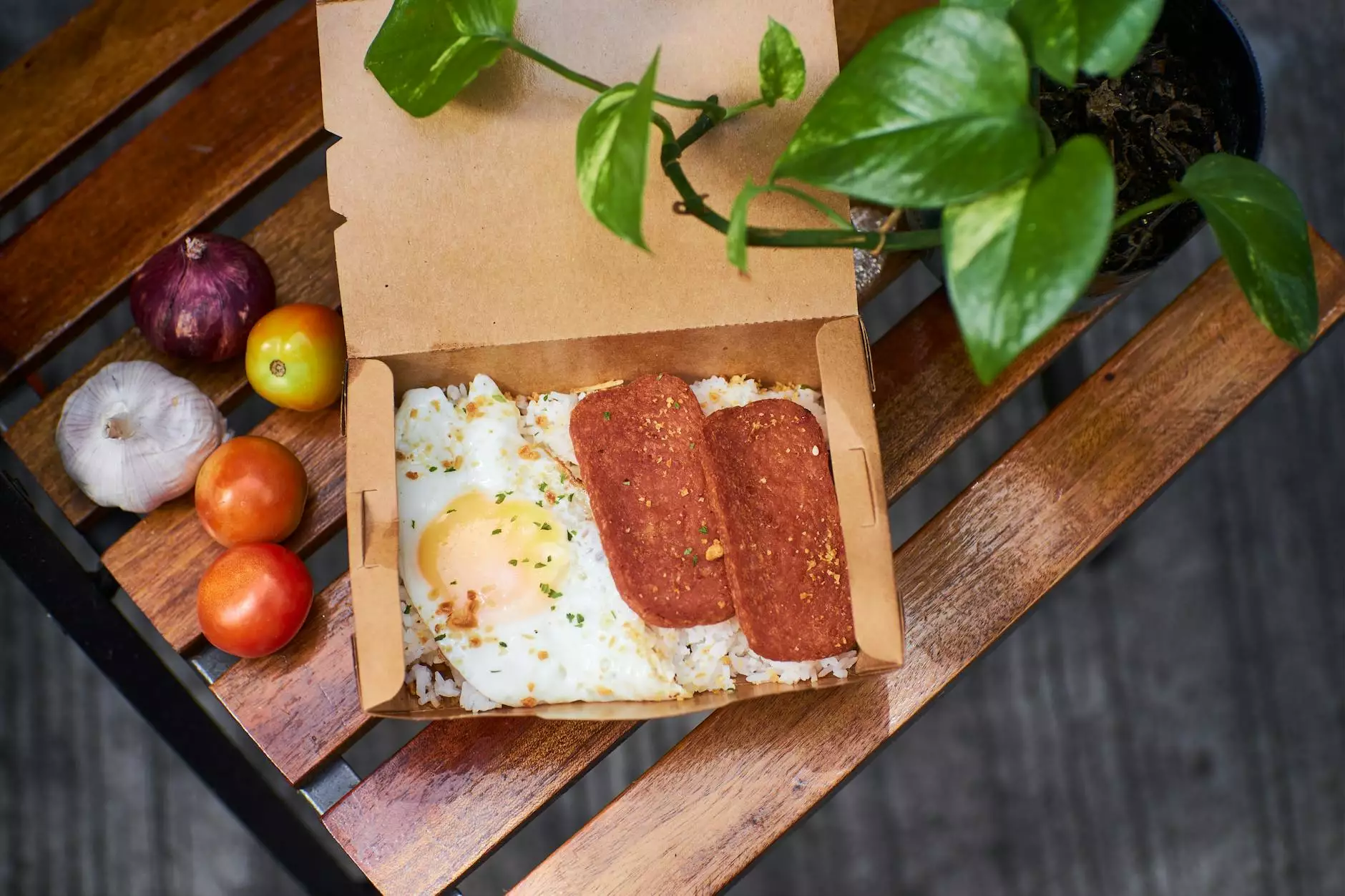
In today's digital age, email has become an essential part of business communication. The rise of email communication has significantly transformed how companies operate, but it has also brought along one major challenge: email spam. Unwanted emails not only clutter inboxes but can also pose serious security threats. Therefore, implementing email spam detection using machine learning has emerged as a crucial solution for businesses seeking to protect their communications and enhance operational efficiency.
Understanding Email Spam: The Threat to Businesses
Before diving into the cutting-edge solutions provided by machine learning, it’s vital to understand the nature of email spam. Email spam refers to unsolicited messages sent in bulk, often for malicious purposes such as phishing, malware distribution, or simply to advertise products or services. This spam can significantly affect a company's productivity and security in several ways:
- Increased Risk of Phishing Attacks: Spam emails often disguise themselves as legitimate communications, tricking employees into revealing sensitive information.
- Loss of Productivity: Employees waste time sorting through spam messages, which can detract from their primary responsibilities.
- Potential Data Breaches: Falling victim to spam can lead to data breaches that damage a company's reputation and finances.
How Machine Learning Revolutionizes Email Spam Detection
Traditional methods of identifying spam often rely on static rules or keyword checking, which can be easily bypassed by savvy spammers. Enter machine learning, a technology that allows systems to learn from data and improve over time. Here’s how machine learning enhances email spam detection:
1. Adaptive Learning Algorithms
Machine learning algorithms can adapt to new spam techniques without requiring manual rule updates. As spammers develop new tactics, the system continues to learn and adjust its detection methods. This dynamic adaptability is crucial in staying ahead of evolving threats.
2. Advanced Pattern Recognition
Machine learning enables advanced pattern recognition, allowing systems to identify subtle indicators of spam emails beyond just keywords. This includes analyzing factors like:
- Sender reputation
- Email formatting and structure
- Behavioral patterns of users interacting with emails
3. Reduced False Positives
One of the significant challenges of email spam detection is minimizing false positives, where legitimate emails are marked as spam. Machine learning models can improve the accuracy of email classification, ensuring that important communications aren't misclassified and missed.
4. Continuous Improvement
Machine learning systems are not static; they continuously improve based on feedback. As users report spam or mistakenly classified emails, the model refines itself, learning from its mistakes to enhance future detection.
Implementing Machine Learning for Email Spam Detection
Implementing email spam detection using machine learning requires a strategic approach. Here are several steps that businesses should consider:
1. Data Collection
Effective machine learning requires relevant data. Start by collecting historical data on emails received within the organization, including a mix of spam and legitimate communications. This data will serve as the training set for the machine learning models.
2. Choosing the Right Algorithm
There are several machine learning algorithms suited for spam detection, including:
- Naive Bayes Classifier: A probabilistic model suitable for large datasets.
- Support Vector Machines (SVM): Effective for high-dimensional spaces.
- Neural Networks: Particularly useful in detecting complex patterns.
The choice of algorithm depends on the specific requirements and available data.
3. Training the Model
Once the algorithm is selected, the next step is to train the model using the collected data. This phase involves feeding the algorithm both spam and non-spam emails so that it can learn to differentiate between the two.
4. Evaluation and Testing
After training, the model must be evaluated against a separate test dataset to measure its performance. Important metrics to consider during this phase include:
- Accuracy: The proportion of true results (both true positives and true negatives).
- Precision: The proportion of true positive results among all positive predictions.
- Recall: The proportion of true positive results among all actual positive instances.
5. Deployment and Monitoring
Once satisfied with the model's performance, it can be deployed within the email system. Continuous monitoring is essential to ensure the model performs as expected in a live environment. This phase includes regular updates and retraining of the model to account for new spam trends.
Benefits of Email Spam Detection Using Machine Learning
The advantages of employing email spam detection using machine learning are multifaceted, greatly benefiting businesses in various ways:
1. Enhanced Security
With robust spam detection, businesses can significantly reduce the risk of falling victim to phishing attacks and malware, securing sensitive data and minimizing breaches.
2. Increased Productivity
By filtering out unwanted emails, employees can focus on priority tasks, leading to improved productivity. An efficient email system enhances workflow and operational efficiency, allowing teams to collaborate more effectively.
3. Reduced IT Costs
Investing in machine learning for spam detection can ultimately lead to decreased IT costs. By preventing spam-related issues, companies can avoid the costs associated with security breaches, data loss, and recovery processes.
4. Improved Customer Communication
A cleaner email environment means that legitimate communications from customers and partners are less likely to be overlooked. This improvement can lead to enhanced relationships and better service provision.
Conclusion
The integration of email spam detection using machine learning stands as a pivotal advancement for businesses seeking to protect their email communications. By leveraging sophisticated algorithms, organizations can efficiently identify spam, reduce risks, and enhance productivity. As cyber threats evolve, investing in advanced solutions like these is not just a choice; it is a necessity for any organization that values security and efficiency. Through platforms like Spambrella, businesses can find the resources and support necessary to implement these cutting-edge technologies and safeguard their digital communications.